GS Yuasa Corporation (Tokyo Stock Exchange: 6674; “GS Yuasa”) and NTT Communications Corporation (“NTT Com”) today announced that the companies succeeded this month in developing AI technology to detect early signs of failure in storage battery systems.
The technology was developed using NTT Com’s AI deep learning-based time-series data analysis technology*1 and lithium-ion storage battery system data from GS Yuasa’s in-house facilities.
1. Background
Lithium-ion batteries are used in a wide range of applications including in equipment used to curb output fluctuations from renewable energy sources such as solar and wind power, backup power sources for electric power and telecommunications facilities, as well as in trains, ships, automated guided vehicles, and other vehicle types. Demand for lithium-ion batteries is expected to increase further as the use of electric vehicles becomes more widespread with the shift toward a carbon-neutral society. At the same time, demand is also growing for technologies to detect early signs of failure in lithium-ion batteries to facilitate safer, more secure, and more stable operation.
It is difficult, however, to foresee potential failures in order to acquire the necessary data in advance, and the threshold values needed to identify failures may vary depending on system operation conditions, thus making suitable criteria adjustments problematic. Further, there remains the issue of system condition diagnoses still being dependent on visual confirmation by highly experienced operators, even as storage battery systems are becoming increasingly large in scale.
To solve these issues, since 2016 GS Yuasa and NTT Com have been working on the development and proof of concept of an AI solution to detect early signs of failure in storage battery systems. With the successful development of such a technology, the companies discovered it was also possible to detect signs of impending failure several months in advance in real-world commercial environments and to distinguish between storage batteries that may experience subsequent failure and storage batteries expected to continue operating normally.
This makes it possible to replace only those storage batteries that may fail in advance of any such potential failure, and also facilitates more efficient monitoring of large-scale storage battery systems while also achieving labor savings, thereby helping to enhance the safety, security, and stability of storage batteries as societal infrastructure.
2. Overview of the new technology
Storage battery system failures are extremely rare, making it difficult to conduct AI deep learning based on data from storage batteries that have actually failed. In cases such as this, one conceivable approach would be to detect abnormal batteries by instead learning about the characteristics particular to normal storage batteries, but this is not easy either given the complexity of the changes in the characteristics of normal storage batteries that occur due to deterioration over time and variations in operating conditions.
The companies’ development work on the new technology therefore involved establishing a method to detect abnormalities utilizing autoencoding*2, a type of unsupervised learning, and developing maintenance-free AI that is able to identify issues in storage batteries despite the complex changes in characteristics that occur even under normal operations.
Development of the technology to detect early signs of failure was made possible by NTT Com’s deep learning-based time-series data analysis technology and lithium-ion storage battery system data from GS Yuasa’s in-house facilities.
3. Evaluation of the newly developed technology
As storage battery failures are so rare, in order to evaluate the new technology GS Yuasa conducted storage battery system feasibility assessments using an in-house ESS (energy storage system) installed with a number of batteries that were modified to generate data with characteristics that differed from the other existing normal storage batteries in the ESS.
The technology avoided detecting erroneous signs of failure from the normal storage batteries while successfully detecting early signs of failure in the modified storage batteries as much as two months in advance of the existing automated alarm system (which alerts operators to failures based on simple automatic evaluations), even when the signs detected did not meet the criteria for failure according to existing definitions.
Further, in tests conducted to verify whether the technology can detect early signs of failure despite changes in the characteristics of normal storage batteries, it was able to identify the modified storage batteries in subsequent evaluations conducted using storage batteries operated under altered conditions.
4. Future developments
GS Yuasa will continue to conduct verification tests to ascertain whether this technology can be utilized in storage battery systems used in a wide variety of different applications. Establishment of a system that can efficiently detect early signs of failure will also help reduce maintenance costs and thereby enable GS Yuasa to further enhance the services it offers to customers.
GS Yuasa will also look at the development of technologies to identify defects in storage batteries through deep learning conducted on data on storage battery failures extracted from the big data collected through remote monitoring systems.
*1 This AI technology is one of the “corevo®” AI technologies of NTT Group. “corevo®” is a registered trademark of Nippon Telegraph and Telephone Corporation.
*2 Autoencoding is a type of machine learning in which algorithms can be used to extract features from data sets through a process of learning to compress and then decompress inputted data.
■Outline of large-scale storage battery system status monitoring
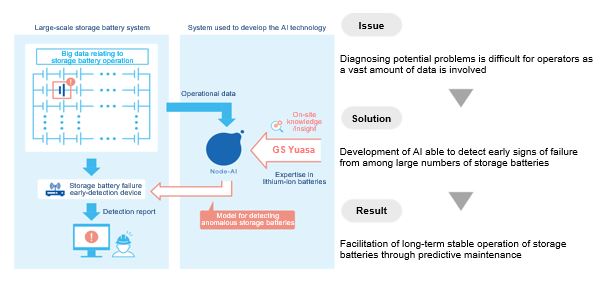
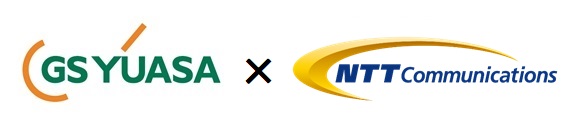